NEODAAS’ artificial intelligence (AI) capabilities are expanding rapidly. Here we are excited to share a new AI video as well as a Q&A with Dr David Moffat, a Artificial Intelligence and Machine Learning Data Scientist at NEODAAS.
Q: Who are you and what do you do?
A: I’m Dr David Moffat and I’m an AI Machine Learning Data Scientist at NEODAAS, hosted by Plymouth Marine Laboratory.
Q: What is AI and how does it try to achieve intelligence?
A: AI is a field of computer science which focuses on trying to build systems which look intelligent. It’s trying to build something that appears intelligent, artificially. We think of human intelligence, so AI tries to make something that behaves in an intelligent way like humans do. A good way to demonstrate this is the Turing test, a test developed by Alan Turing in the 1950s, whereby written instructions are sent to a computer and a human. If in the responses the computer cannot be identified from the human then the computer has passed the Turing test and can be considered artificially intelligent.
Q: How long has AI been around and why is it advancing now?
A: AI has been around for decades; a lot of the Machine Learning methods we use today were invented back in the 70s, 80s and 90s. It’s advancing now because we finally have enough computational power and data to take full advantage of them, especially with deep learning and neural networks.
Q: What is machine learning and how does it relate to AI?
A: Machine learning is a specific field within AI. It tries to build something that looks intelligent by learning from data. So you feed in lots of data and the system tries to learn what intelligent behaviour would be from that data. This is why machine learning is key right now, because we have huge datasets that can be used for learning.
Q: Can you explain deep learning and neural networks?
A: Neural Networks are a machine learning method, which have series of `neurons’ connected together, based on our understanding of how the brain works with interconnected neurons sending signals back and forth. Deep learning uses these neural network structure, in which you have many layers of neurons, sometimes called ‘hidden layers’ These layers of neurons can model more complex functions. The more layers, the “deeper” the machine learning model.
Q: How does PML use AI and machine learning with all the data you gather?
A: We leverage machine learning to process huge amounts of data and try to extract meaning from it. This could be identifying features in satellite images, detecting species in drone footage, or interpreting data to identify relationships. At PML we have petabytes of satellite data and do lots of field sampling and surveys so the data we hold just keeps growing.
Q: What types of data do you work with daily at PML?
A: I primarily work with satellite data from the European Space Agency (ESA) and the National Aeronautics and Space Administration (NASA) to understand what’s happening with the oceans. We also do field work like drone flights, putting cameras on ships, taking water samples from research boats and measuring water conditions via data buoys.
Q: Can you give an example project where you’ve used AI at PML?
A: We predicted harmful algal blooms by matching up satellite images with where people had sampled harmful algal species. We trained a model to detect these blooms, which is useful because harmful algal blooms can have a negative impact on aquaculture and make shellfish dangerous for humans.
Q: How long has PML been studying algal blooms and how does AI advance this?
A: PML has studied algal blooms for decades by looking for colour signatures in optical satellite images. Now with AI we can build more complex models incorporating other data like temperature, chlorophyll levels, etc. to try and detect different species, and to predict when and where they will occur.
Q: For the algal bloom project, did you build custom models or use pre-built algorithms?
A: For that project we built custom neural networks from scratch, gathering the satellite data and training models specifically to identify harmful blooms. For other projects, like identifying Pacific oysters in drone images, we have used off-the-shelf algorithms like YOLO and fine-tuned them for our particular data.
Q: Can you describe the Pacific oyster project?
A: We are training machine learning models to automatically detect invasive Pacific oysters along the Devon coast from drone imagery. It used to require manual counting of oysters across sites, which was resource intensive. On this project, my colleagues went out and did some drone flights over a series of sites where these oysters have been spotted and then another one of my colleagues sat down and manually annotated and identified about 7,000 oysters. It’s quite time consuming work. Now that the algorithm has been trained, AI can identify and count them in a fraction of the time, making it much easier to monitor.
Above: Photos from the Pacific Oyster project. 1st photo shows Silvia Almeida (a PhD student based at MARE in Portugal, that came to PML to collaborate on this fieldwork as part of PORTWIMS) operating a drone for fieldwork. The 2nd photo shows our Earth Observation Scientist and Drone Captain Aser Mata, who led the collection of drone footage during fieldwork for the project, with one of the Pacific Oysters. The 3rd photo shows Pacific Oysters captured as part of the fieldwork, next to an image on the right showing the AI detection of oysters – with green dots on the corners of each automatically detected oyster. Image credits: Aser Mata.
Q: What hardware does PML use to run machine learning models?
A: We have a graphics processing unit (GPU)-based high-performance computing cluster called MAGEO (MAssive GPU cluster for Earth Observation) specifically built for AI. The GPUs we use are optimized for running deep neural networks so they enable us to train state-of-the-art machine learning models. MAGEO is operated as part of the NEODAAS AI service and is available to all UK researchers through Natural Environment Research Council’s (NERC) funding.
Q: What makes PML an exciting place to work on AI right now?
A: PML is an organisation that has a long history of collecting and analysing environmental data and has staff with decades of experience doing this. Recent innovations in data collection, such as automatous vessels and Earth Observation, including drones, mean we now have more data than ever before. So much data, in fact, it is more than the experts at PML can use. This presents a big opportunity to apply AI to analyse these data being collected across scientific domains and disrupt traditional techniques. PML has an extensive track record of engaging with international policy so the work on AI will help inform policies that aim to improve the state of our oceans.
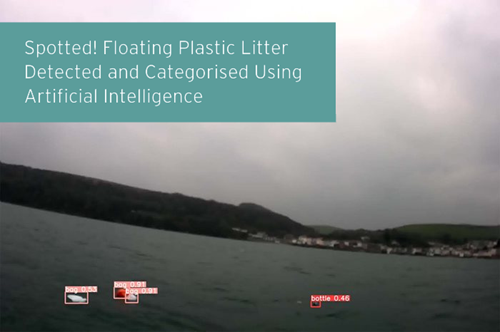
Q: What technical considerations is most important when applying AI?
A: It’s crucial to ensure the problem is actually suited for AI in the first place. You need to understand the problem you are trying to solve and how the AI model will be able to help rather than simply throwing any AI algorithm at some data. The pacific oyster detection is a good example of this as we know that pacific oysters look very distinct in images from drones. Writing a set of rules to explain this (e.g. pacific oysters are white and have a specific texture) is difficult so it is a good candidate to make use of AI and learn from a set of examples. Having determined that it is suitable for applying AI, we know an algorithm is needed that is very good at identifying objects in images, and there is an existing algorithm (YOLO) that does this. We also know that YOLO was not designed for detecting pacific oysters in drone imagery as this is a very specific application, so we need to train it to recognise them. We go through a similar thought process with every problem for which we are thinking of using AI.
Q: What ethical concerns need to be addressed with AI?
A: You must recognize biases inherent in data that propagate through AI models, especially if they will impact people’s lives. We start by considering ethics from the beginning, not an afterthought. Our models are held to the same integrity standards as all PML science – we carefully interrogate data/methodology and apply rigorous validation procedures for trustworthy, unbiased techniques.
Q: How can AI specifically help advance environmental sciences?
A: AI is great for extracting complex meaning from complex data, from species in drone images to better monitoring of biodiversity. We can also build models of environments to determine drivers and relationships between components. We’ve done some recent work where we’ve looked at plankton in the oceans and how much chlorophyll and carbon they have so we can identify the environmental drivers that are impacting them in different geographical regions, to try and understand what in the system is driving it. Overall it gives us a deeper understanding of the environmental processes.
Q: How do you address the carbon footprint of AI at PML?
A: We’ve installed solar panels to help offset the compute cost of training models. We also prototype on smaller datasets first to rapidly iterate, only scaling up later once we know models work to avoid unnecessary emissions. And we have specific models of power efficient GPUs to reduce our power consumption.
Q: Is PML’s AI infrastructure available for other researchers?
A: Yes, through the AI service operated as part of NEODAAS, other UK academics can apply to access PML’s MAGEO supercomputer and collaborate with PML’s AI experts for their own machine learning projects related to the natural environment. We are also founding members of Smart Sound Plymouth, a pioneering technology development platform facilitating the design, testing and development of new products and services for the marine sector. Through Smart Sound Plymouth, uses can access to the UK’s premier proving area for emergent cutting edge marine technologies that includes AI capabilities, to enhance data processing and gain greater insight for testing.
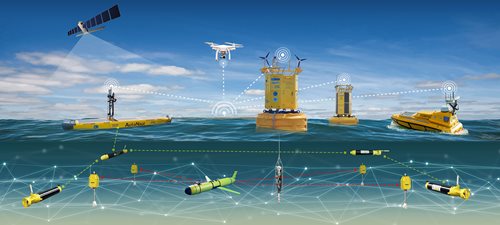
Q: What most excites you about the future potential for AI?
A: AI is an extremely powerful tool for analysing all the data we constantly collect about our planet and climate. It can help us answer fundamental questions about what’s happening to our environment. Understanding and addressing climate change is humanity’s great challenge and AI is key.
Q: How do you see AI progressing in the coming years?
A: AI will keep advancing rapidly but may be slower than many predict. It’s taken decades to reach this point already and we still have major barriers to overcome. It will become more integrated in daily life but it already has been for years e.g. autofocus, self-driving cars. It augments human capabilities rather than replacing them. I believe in using AI to automate mundane tasks, or as a supportive collaborative tool for more creative tasks. There’s been a lot of question recently around computers and AI having some sort of creativity. Whether AI can generate music and generate songs and things like that, that’s a whole other topic of conversation but there will always be a place for a person within the context of AI. You still need someone to use the tools, to drive it forward and provide human oversight.
Further Information
NEODAAS Artificial Intelligence Service
Artificial intelligence and machine learning at Plymouth Marine Laboratory